Years ago, editor of an important U.S. agricultural journal showed me a manuscript he was going to reject, only because it was about applications of “artificial neural networks” in agriculture. He was apparently sick of receiving so many papers about ANNs all sent by authors from a developing country. At the time, nobody in our department was interested in machine learning. Ironically, a few weeks ago I received a manuscript about the same topic for review co-authored by the same person.
These days, almost any manuscript (research paper) I receive for review is focused on the applications of machine or deep learning in agriculture. What is new, however, is so many submissions by computer scientists or electrical engineers in agricultural topics. Even though I appreciate the enthusiasm, and no offense to the aforementioned group, I rarely find anything of value for the agricultural community in these types of papers. I cannot understand why someone with mere expertise in programming and machine learning might think they have all the answers in a field like agriculture!?
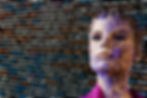
They are not the only ones to blame. Apparently these days, the trend is to kick talents out of the field and bring in non-experts instead. Agricultural and biological engineering departments are showing more and more interest in hiring graduates with background in computer science because of their extensive knowledge of artificial intelligence (AI). At the same time, companies like Amazon, Facebook and even major financial institutions are hiring agricultural and biosystems engineers as data scientists to work on their fraud detection, etc. projects using machine learning. These companies offer salaries that no company in the agricultural industry can match, so we are losing more and more talents to other industries every day. I know at least two agricultural graduates with PhD who currently work for banks and one who now uses their expertise in medical imaging.
Let's be honest here. If I'm a biosystems engineer with expertise in computer vision and AI, why would I want to work a job in agriculture which pays me half the normal salary in other industries??
There is a saying among AI experts that “AI is only half the solution”. We cannot ignore the importance of data and hardware that is often needed to collect data. Companies like Google and Facebook collected customer data for many years and built their AI algorithms on the top of a huge amount of data (big data). According to statistics, only 11% of the U.S. agriculture has been exposed to sensor technologies. Most farmers are comfortable with not using any form of technology for water, nutrients or pest and disease management. So, there is a huge gap in agriculture in terms of data. While there are free data and databases available on the Internet for people to use, they are mostly unusable for agricultural applications. If one cannot take advantage of “transfer learning”, it means they need much more data and much more time to develop a functional AI model with acceptable accuracy.
Considering where we are now with the AI technology, can IoT-enabled wireless sensor networks, imaging systems and fancy AI models replace farmer's intuition?
Another issue is that, people (usually non-AI experts) are hoping to achieve magical results using AI while they are using unreliable sensors and/or fusing sensor data that are logically unrelated. The process of observing and analyzing (in one’s mind) is gradually being replaced by the “let’s throw everything into the [AI] algorithm and see what happens” approach, as if AI is some alien technology that is far superior to ours.
Assuming that AI is a technology that we can rely on, we first need to work on the infrastructure that is required to implement it in agriculture. There are many issues to solve first like technology adoption by growers, sensor accuracy, data collection in remote areas and connecting farms to the Internet. There are important environmental parameters that we do not even have sensors to measure yet.

Other than to say, we have not been able to address issues as obvious as water shortage in agriculture using reliable methods that have evolved over decades. How on earth we will be able to solve problems using a “black box” (AI)?
We have not been able to convince growers to invest in sensors yet. How on earth are we going to be able to convince them to buy sensors plus everything else that is needed to run AI?
My other concern is the kind of biosystems engineers we are currently training at universities if they will be spending four years sitting in front of computers annotating images and running codes. A major difference that agriculture has with many other industries is the fact that we need to have our hands dirty in the field in order to learn about the system. Have we already learned all we needed about agriculture that now we can just sit in our offices and rely on our field setups?
I am worried we might get to a point in five to ten years where we finally realize we need more than just “semantic image segmentation” to solve our problems in agriculture, but by then the damage to the field might be beyond repair.
To summarize, this is what is happening in agriculture with respect to AI in my opinion:
In most cases, we develop machine learning-based solutions with no intention to solve existing problems, but to get funded, publish papers and get promoted.
We are losing talents to other industries and bringing in non-experts into agriculture.
We are not training agricultural engineers who are problem solvers, we are training fancy programmers with PhDs.
We are relying on AI as if it is an alien technology capable of solving all our problems.
We are ignoring the infrastructure required for AI implementation in agriculture.
---------------------------------------------------
Here's parts of the best article I've read so far about "Artificial Intelligence". I strongly recommend it:
Dr. Melanie Mitchell, Davis Professor of Complexity at Santa Fe Institute
"... In 1892, the psychologist William James said of psychology at the time, “This is no science; it is only the hope of a science” [84]. This is a perfect characterization of today’s AI. Indeed, several researchers have made analogies between AI and the medieval practice of alchemy. In 1977, AI researcher Terry Winograd wrote, “In some ways [AI] is akin to medieval alchemy. We are at the stage of pouring together different combinations of substances and seeing what happens, not yet having developed satisfactory theories...but...it was the practical experience and curiosity of the alchemists which provided the wealth of data from which a scientific theory of chemistry could be developed” [85]. Four decades later, Eric Horvitz, director of Microsoft Research, concurred: “Right now, what we are doing is not a science but a kind of alchemy” [86]. In order to understand the nature of true progress in AI, and in particular, why it is harder than we think, we need to move from alchemy to developing a scientific understanding of intelligence. "