Use of AI and Computer Vision in Indoor Farming: Misconceptions
- Y. Osroosh, Ph.D.
- May 10, 2024
- 3 min read
Updated: Apr 21
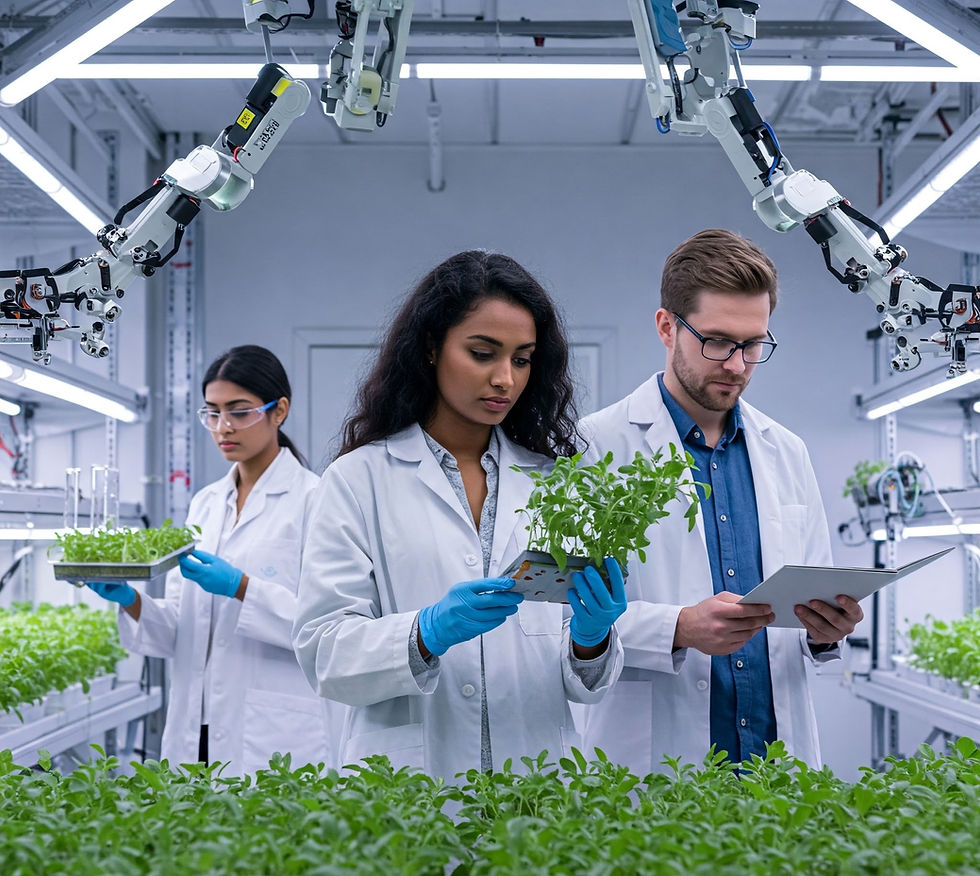
It caught my eye the other day: "AI-enhanced indoor farming takes root at the University of..." The news piece elaborated, stating that researchers at the university's Institute for Artificial Intelligence and Data Science are cultivating plants under an "Artificial Intelligence system that is designed to identify any signs of sickness or distress in the plants." It's certainly encouraging to see institutions, even those without traditional agricultural colleges, engaging in research relevant to food production.
Indeed, the potential of AI and computer vision to revolutionize agriculture is undeniable. However, what often concerns me is the apparent disconnect between the sophisticated tools being developed and a fundamental understanding of the agricultural domain they are intended to serve. It's as if the excitement surrounding AI overshadows crucial biological and environmental realities.
Time and again, I encounter similar oversights in studies exploring the application of these technologies in agriculture (and not necessarily limited to the aforementioned university's work). Two common examples immediately come to mind: the misapplication of the Normalized Difference Vegetation Index (NDVI) for water stress detection and the confusion between Near-Infrared (NIR) and Thermal Infrared (IR) radiation.
While I won't delve into exhaustive technical explanations here, it's crucial to highlight a few key points that researchers and enthusiasts in this field should internalize:
NDVI: A Broad Stroke, Not a Fine-Tuned Water Management Tool: NDVI, derived from multispectral or hyperspectral imagery, excels at distinguishing between healthy, actively growing vegetation and dead or severely stressed plants. However, its sensitivity to subtle changes in plant water status is limited. Therefore, attempting to correlate NDVI directly with soil water content for precise irrigation management is often a futile exercise. It's a valuable indicator of overall plant health, but not a reliable metric for day-to-day water decisions.
Thermal Imaging Holds the Key to Water Stress Detection: Conversely, normalized thermal data, often expressed as the Crop Water Stress Index (CWSI), offers a much more direct and sensitive measure of plant water stress. By capturing the thermal radiation emitted by plants, CWSI can detect even mild levels of water deficit, making it a valuable tool for timely irrigation scheduling. When coupled with appropriate models, CWSI can exhibit a strong correlation with soil water deficit.
Holistic Monitoring: Biotic and Abiotic Stresses Intertwined: In the controlled environment agriculture (CEA) setting, it's unrealistic to isolate biotic (e.g., pests, diseases) or abiotic (e.g., water stress, nutrient deficiencies) stresses. Often, the visual symptoms of these different stressors can be remarkably similar. Effective monitoring requires a comprehensive approach, employing a suite of relevant sensors to simultaneously track various plant health indicators.
Plant Physiology Dictates, Algorithms Follow: There are inherent biological limits to how much we can manipulate plant responses simply by adjusting environmental factors like the light source. Ultimately, our AI systems and algorithms must be designed to adapt to the plants' needs and natural rhythms, not the other way around. The plant's stage of development and even the timing of measurements can significantly influence the data we collect and the insights we derive.
AI: Not a Substitute for Agricultural Expertise and Established Models: The allure of the "black box" AI/ML/DL approach can be strong, particularly for data scientists lacking deep domain expertise in agriculture. However, established theoretical crop models, developed through decades of research, offer robust frameworks that hold true across various conditions. Ideally, a more effective approach involves integrating these well-validated models with the pattern recognition capabilities of AI to create truly resilient and insightful agricultural tools. An AI model that performs flawlessly in a controlled lab setting may very well falter in a real-world CEA environment if crucial agricultural factors are overlooked.
Garbage In, Garbage Out: The Limits of AI Magic: There's a misconception, which frankly baffles me, that AI possesses some magical ability to compensate for poor-quality sensor data. The reality is starkly different. AI models are built upon the data they are fed. If your initial sensor data is noisy, inaccurate, or doesn't capture the relevant information, the resulting AI model will inevitably be flawed. If your standard RGB camera can't detect a subtle plant anomaly, no amount of sophisticated AI processing will magically reveal it.
This isn't to diminish the immense potential of AI and computer vision in indoor farming. However, it's a plea for a more grounded and interdisciplinary approach. We must bridge the gap between cutting-edge technology and fundamental agricultural knowledge to truly unlock the benefits of these powerful tools.
For a deeper exploration of the potential pitfalls of the uncritical application of artificial intelligence in agriculture, I strongly encourage you to read my previous post on "How AI Research is Misguidedly Transforming Agricultural Innovation." Understanding the potential downsides is just as crucial as embracing the possibilities.
Comments